Nu10 Insights
Practitioners/Doctors
Comparing Leading AI Models for Clinical Text Processing: An Observational Study
Want to Discuss more?
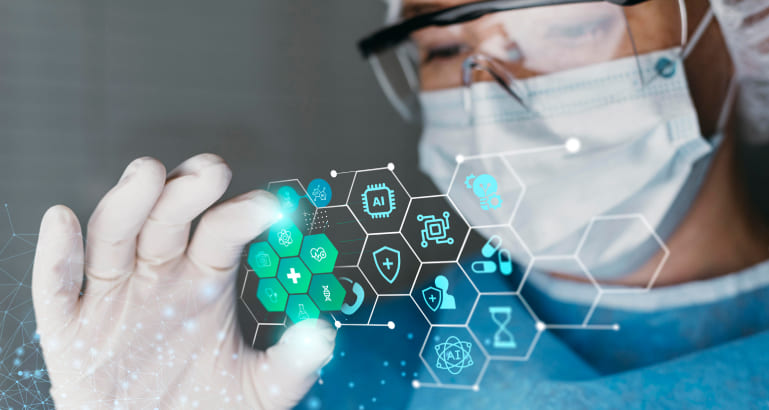
Abstract
The burgeoning volume of clinical documentation presents a significant challenge in modern healthcare, often diverting clinicians' attention from direct patient care [1]. This study evaluates the potential of three leading artificial intelligence models: ChatGPT, Claude, and Gemini, to alleviate the clinical documentation burden. We assessed these models on data extraction, data analysis, and document summarization tasks using a small set of diverse clinical texts: radiology reports, patient histories, and clinical notes. Our evaluation aims to reveal notable differences in model performance across tasks and text types. This study provides insights into the current state of AI-assisted clinical text processing and its potential to support healthcare professionals.
Introduction
The digital transformation of healthcare has brought with it an unintended consequence: an unprecedented increase in documentation requirements. Electronic Health Records (EHRs), while improving accessibility and continuity of care, have significantly expanded the administrative workload for clinicians [2]. Recent studies indicate that physicians now spend up to two hours on documentation for every hour of direct patient interaction, while nurses may dedicate up to 60% of their time to documentation tasks [3]. This documentation burden not only contributes to clinician burnout but also potentially compromises patient care by reducing face-to-face interaction time [4].
Artificial Intelligence (AI), particularly in the form of large language models (LLMs), has emerged as a potential solution to this challenge. These models have demonstrated remarkable capabilities in natural language processing tasks, including text summarization, information extraction, and analysis [5]. However, the application of these models in the highly specialized and sensitive domain of healthcare requires careful evaluation.
In this study, we focus on three leading AI models: GPT, Claude, and Gemini [6, 7, 8].Our goal is to assess and compare the performance of these models on three critical clinical text-processing tasks:
- Data Extraction: The ability to identify and extract specific pieces of information from clinical texts.
- Data Analysis: The capacity to interpret clinical data and provide insights or conclusions.
- Document Summarization: The skill of condensing lengthy clinical documents into concise, informative summaries.
We evaluate these tasks across three types of clinical text:
- Radiology Reports: Often structured but containing specialized terminology.
- Patient Histories: Typically narrative in nature, with varying levels of detail and organization.
- Clinical Notes: Usually more technical, containing a mix of objective observations and subjective assessments.
By examining the performance of ChatGPT, Claude, and Gemini across these diverse tasks and text types, we aim to provide a comprehensive understanding of their current capabilities and limitations in processing clinical text. This evaluation is crucial for assessing the potential of these AI models to alleviate the documentation burden on healthcare professionals while maintaining or improving the quality of clinical information management.
Methods
Data Collection and Preparation
We selected a small dataset of 5 samples each of radiology reports, patient histories, and clinical notes. All data was de-identified to protect patient privacy.
Model Setup
In this setup, we focus on particular versions of three leading AI models: ChatGPT 3.5, Claude 3.5 Sonnet, and Gemini 1.5 Flash. These are the free available versions of each model at the time of writing this article. Each of these models represents a different approach to AI development:
- ChatGPT 3.5. developed by OpenAI, is known for its broad knowledge base and versatility across various tasks [6].
- Claude 3.5 Sonnet, created by Anthropic, is designed with a focus on safety, ethical considerations, and a huge context window of 200,000 tokens [7].
- Gemini 1.5 Flash, Google's latest AI model, is praised for its multimodal capabilities and efficiency [8].
For each model, we developed task-specific prompts that included clear instructions and, where applicable, examples of desired outputs. We did not perform any fine-tuning on the models to assess their out-of-the-box performance on clinical tasks. The temperature and all the other settings were set to default as well.
Tasks
- Data Extraction: Models were tasked with extracting key clinical information such as diagnoses, medications, vital signs, and test results from the texts.
- Data Analysis: Models were asked to interpret clinical data, identify trends, and suggest potential diagnoses or treatment considerations based on the information provided.
- Document Summarization: Models were instructed to create concise summaries of the clinical texts, highlighting the most important information.
Evaluation Criteria
We assessed the models' performance based on the following key criteria:
- Precision: The percentage of correctly identified relevant instances out of all instances identified by the model. High precision indicates that the model has a low false-positive rate[9].
- Recall (Sensitivity): The percentage of correctly identified relevant instances out of all actual relevant instances. High recall indicates that the model has a low false-negative rate [10].
- Completeness: The degree to which all necessary and relevant information is accurately captured and addressed by the model [11].
- Clinical Relevance: The significance and applicability of the information or insights provided, ensuring they are meaningful in a clinical context.
- Conciseness: The model's ability to convey information efficiently, avoiding unnecessary details while maintaining clarity [12].
Evaluation Process
We performed a detailed qualitative analysis of cases where models performed particularly well or poorly to identify patterns and potential areas for improvement and compare them with each other against the evaluation criteria to conclude which AI model is a better fit for clinical documents varying in types, sizes, and the kind of information.
Results
Data Extraction
Criteria | ChatGPT 3.5 | Claude 3.5 Sonnet | Gemini 1.5 Flash |
---|---|---|---|
Precision | Medium | High | Medium |
Recall | Medium | High | Low |
Completeness | High | High | Medium |
Clinical Relevance | High | High | Medium |
Conciseness | Medium | High | Low |
ChatGPT 3.5 performed comparably better than Gemini in structured data extraction. It showed particular strength in maintaining consistency across similar data points. For instance, in extracting medication information from clinical notes, GPT was more likely to maintain a consistent format (e.g., always including dosage and frequency) even when this information was presented inconsistently in the source text. Its recall was moderate, occasionally missing some relevant details, but it maintained high completeness by capturing the most necessary information.
Claude 3.5 Sonnet excelled particularly in extracting complex medical terms and interpreting ambiguous phrasing. For example, in a radiology report stating "ground-glass opacities in the right upper lobe,” and “Nonspecific patchy ground-glass opacification and septal thickening in bilateral upper lobes" Claude correctly extracted both the finding (ground-glass opacities) and the location (right upper lobe), while also noting the uncertainty regarding infection. Claude's completeness was notable, thoroughly covering necessary details with high clinical relevance. It was also highly concise, efficiently presenting information without unnecessary elaboration.
Gemini 1.5 Flash while slightly behind GPT and Claude in overall precision, demonstrated better performance in extracting numerical data and units upfront, particularly from lab results within clinical notes. For example, Gemini consistently extracted and correctly interpreted lab values like "Lopressor 50 mg PO tabs 1 tab twice a day" including contextual information about the reference range. Its clinical relevance was moderate, sometimes focusing on less important details, and it tended to be less concise, often providing more information than necessary.
Data Analysis
In data analysis tasks, the performance differences between the models were more pronounced and varied depending on the type of clinical text.
Criteria | ChatGPT 3.5 | Claude 3.5 Sonnet | Gemini 1.5 Flash |
---|---|---|---|
Precision | High | High | Medium |
Recall | Medium | High | Medium |
Completeness | Medium | High | High |
Clinical Relevance | High | High | Medium |
Conciseness | Medium | Medium | Low |
For radiology reports, Claude 3.5 Sonnet outperformed the other models, demonstrating a fine understanding of radiological findings, patient histories, and their clinical implications. In one notable example, Claude correctly identified a case of COPD with potential chronic inflammation based on a combination of imaging findings and patient history, suggesting appropriate follow-up steps. Claude's completeness was high, covering a wide range of clinical implications, though its analyses could sometimes be lengthy despite their high clinical relevance.
ChatGPT 3.5 excelled in analyzing patient histories, showing a strong ability to integrate information from different parts of the narrative to form cohesive clinical understanding. For instance, in a complex case involving a patient with multiple chronic conditions, GPT accurately highlighted potential drug interactions and suggested adjustments to the treatment plan. However, Claude's clinical relevance for patient history analysis was observed to be higher overall. Its completeness was moderate, occasionally missing nuanced details, but it maintained high clinical relevance, especially in patient histories.
Gemini 1.5 Flash demonstrated particular strength in analyzing quantitative data, showing high completeness in this area. However, its overall precision and recall in data analysis were moderate, with some inaccuracies in interpretations and missed clinically relevant patterns. Gemini's clinical relevance was medium, sometimes focusing on less critical aspects.
Document Summarization
In summarization tasks, Claude outperformed the rest of the models, but each had their distinct characteristics:
Criteria | ChatGPT 3.5 | Claude 3.5 Sonnet | Gemini 1.5 Flash |
---|---|---|---|
Precision | High | High | Medium |
Recall | Medium | High | Medium |
Completeness | High | High | High |
Clinical Relevance | High | Medium | Medium |
Conciseness | Medium | High | Low |
ChatGPT 3.5’s summaries were consistent most of the time, striking an impressive balance between conciseness and informativeness. GPT's ability to prioritize information, consistently highlighting the most clinically relevant points at the beginning of each summary was very useful. GPT's clinical relevance in summaries was medium, sometimes overlooking subtle clinical implications. It struck an impressive balance between conciseness and informativeness.
Gemini 1.5 Flash’s summaries were noted for their clear structure and readability, often using bullet points or subheadings to organize information effectively. This was particularly evident in summarizing lengthy clinical notes, where Gemini's structured approach could make it easy for clinicians to quickly grasp key points. However, its precision was medium, with some inaccuracies in summaries and often missing some important details.
Discussion
Our evaluation of ChatGPT 3.5, Claude 3.5 Sonnet, and Gemini 1.5 Flash on clinical text processing tasks reveals that these AI models have achieved a level of performance that could significantly impact healthcare documentation practices [13]. Each model demonstrated unique strengths:
- ChatGPT 3.5 consistently provided concise, narrative-style responses that captured key information efficiently. Its summaries were particularly reader-friendly and resembled natural language used by clinicians.
- Claude 3.5 Sonnet excelled in providing structured responses, clearly categorizing information and often separating positive and negative findings. This approach could be particularly useful for quick information retrieval in clinical settings. Moreover, Claude 3.5 Sonnet's exceptional context window of 200,000 tokens allows it to process and analyze much larger volumes of clinical text in a single interaction, making it particularly suitable for handling comprehensive medical records or multiple documents simultaneously.
- Gemini 1.5 Flash demonstrated a tendency to provide the most comprehensive and detailed responses, often including aspects not mentioned by the other models. Its use of numbered lists and subsections could be beneficial for complex cases requiring detailed documentation.
General Model Characteristics
Characteristic | ChatGPT 3.5 | Claude 3.5 Sonnet | Gemini 1.5 Flash |
---|---|---|---|
Context Window | 16k tokens | 200k tokens | 128k tokens |
Parameter Size | 175 billion | 2 trillion + (estimated) | 1.6 trillion (estimated) |
Quality | High | High | Medium |
Bias Mitigation | Medium | High | Medium |
Inference Speed | High | High | Medium |
Notably, Gemini 1.5 Flash frequently defaulted to a generic 'I am only an AI model' response, failing to complete tasks despite identical prompts. This limitation necessitated API workarounds, significantly hindering our progress.
In contrast, ChatGPT 3.5 and Claude 3.5 Sonnet demonstrated consistency. However, ChatGPT 3.5's constrained context window posed significant limitations. Therefore, Claude 3.5 Sonnet's capabilities shone, particularly in clinical text processing and document summarization, making it our preferred choice for these applications.
Key Observations
- Data Extraction: All models precisely extracted key information from radiology reports but with varying styles of presentation. Claude 3.5 Sonnet and Gemini 1.5 Flash's structured formats might be preferable for quick data review.
- Data Analysis: The models showed different strengths in analyzing patient histories. GPT-3.5 provided concise, actionable insights; Claude 3.5 Sonnet offered broader differentials and treatment considerations while ChatGPT 3.5 included a more comprehensive workup.
- Document Summarization: In summarizing clinical notes, all models captured the essential information, but Claude 3.5 Sonnet’s detailed, structured approach stood out for its comprehensiveness and relevance, while ChatGPT 3.5’s was more concise while maintaining structure.
Notably, each AI model exhibited distinct approaches to structuring and presenting information. Despite similarities in content and context, conciseness, clinical relevance, and precision variations influenced our preference for one model over others.
Model Comparison
- Claude 3.5 Sonnet emerged as the top choice, excelling in most cases, thanks to its expansive context window and exceptional document information extraction capabilities.
- ChatGPT 3.5 closely followed, demonstrating strong performance with structure and clinical relevance.
- Gemini 1.5 Flash trailed behind, despite its potential.
Claude's superior performance was largely attributed to its ability to process extensive context and extract relevant information from documents, making it an ideal choice for clinical text processing and summarization.
Important Considerations
However, several important considerations emerged from our study:
- Clinical Relevance: While all models performed well, there were instances where they did include irrelevant information sometimes or missed subtle but clinically important details. This highlights the continued need for human oversight and the importance of domain-specific training or fine-tuning.
- Consistency: We observed some variability in performance across different samples, particularly in more complex cases. Ensuring consistent reliability across diverse clinical scenarios remains a challenge.
- ExplainabilityThe 'black box' nature of these models' decision-making processes raises questions about their interpretability and accountability in clinical settings.
- Privacy and Security: Although this study used de-identified data, the deployment of such models in real-world clinical settings would require robust safeguards to protect patient information.
- Integration with Clinical Workflow: The practical implementation of these models would require careful consideration of how they integrate with existing EHR systems and clinical processes.
Limitations
Our study, while comprehensive, has several limitations. The dataset, though diverse, was from a single institution and may not fully represent the variety of documentation styles and clinical contexts across different healthcare settings. Additionally, the evaluation was based on the models' performance at a single point in time, and given the rapid pace of AI development, these results may quickly become outdated as models only get better.
Conclusion
Our study demonstrates that leading AI models like ChatGPT 3.5, Claude 3.5 Sonnet, and Gemini 1.5 Flash have achieved a level of proficiency in clinical text processing that could significantly alleviate the documentation burden on healthcare professionals. Each model showed distinct strengths across various tasks and evaluation criteria.
While all models performed well, Claude 3.5 Sonnet's combination of high precision, clinical relevance, and an exceptionally large context window of 200,000 tokens positions it as a particularly promising tool for processing extensive medical records and complex clinical documents. This capability could be especially valuable in real-world healthcare settings where comprehensive patient histories and large volumes of clinical data need to be analyzed simultaneously. Claude seems to be the logical choice here when choosing an AI model to productionize clinical text processing tasks on a large scale.
However, it is crucial to emphasize that these AI tools are best viewed as supportive technologies to augment, rather than replace, clinical expertise. The nuanced nature of medical decision-making, the critical importance of context in patient care, and the ethical considerations surrounding healthcare delivery necessitate continued human oversight and involvement [14].
The integration of these AI models into clinical workflows holds the promise of not only reducing administrative burden but also potentially improving the quality and consistency of clinical documentation. This could lead to more efficient healthcare delivery, allowing clinicians to dedicate more time to direct patient care and complex decision-making tasks.
References
- Measurement of clinical documentation burden among physicians and nurses using electronic health records: a scoping review | Journal of the American Medical Informatics Association | Oxford Academic
- System-Level Factors and Time Spent on Electronic Health Records by Primary Care Physicians | Artificial Intelligence | JAMA Network Open
- Tethered to the EHR: Primary Care Physician Workload Assessment Using EHR Event Log Data and Time-Motion Observations | Annals of Family Medicine
- The Burden and Burnout in Documenting Patient Care: An Integrative Literature Review
- Recent Advances in Generative AI and Large Language Models: Current Status, Challenges, and Perspectives
- ChatGPT
- Claude
- Gemini
- Precision in Machine Learning.
- Classification: Accuracy, recall, precision, and related metrics | Machine Learning | Google for Developers
- AI-complete | AI Glossary
- How to Write Clearly with Generative AI
- How AI Improves Healthcare Documentation | Uptech
- ACP recommends AI tech should augment physician decision-making, not replace it
About Author

Mohit Kataria
Mohit is a tech-enthusiasts who have founded and built Manthan Research and Analytics, which got acquired by M3 and got rebranded to m360 Research. M3 is a $40bn+ Japanese Medical Information firm which is the youngest Nikkei 225 company. During his tenure there, it specialized in using cutting-edge advanced analytics techniques like NLP, unstructured data analytics, machine learning to drive actionable intelligence. He enjoys breaking new ground, building & scaling high-performing teams ground up, and creating new solutions.